Growing Your Own Data Scientists
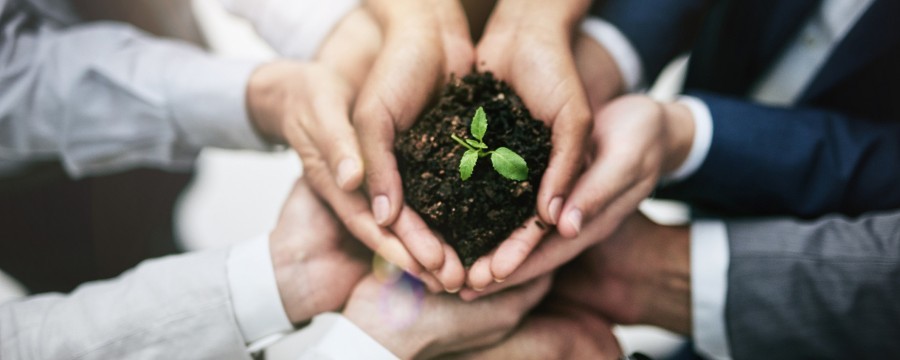
CIOs and CTOs must learn to address a challenge, involving the divide between the people who know about the vast amount of new sources of data emanating from machines and other devices (“big data”) and the questions in the enterprise whose answers can be monetized.
CIOs and CTOs must learn to address a challenge, involving the divide between the people who know about the vast amount of new sources of data emanating from machines and other devices (“big data”) and the questions in the enterprise whose answers can be monetized. One group of people knows about the technology for analyzing data (they’re usually in IT).
The other group understands the pernicious questions that would lead to an answer that is worth money to an organization (they’re usually on the business side). The role of the data scientist is a hybrid role that can solve this problem.
While the definition of the role is compelling, it’s a lot easier to define the role than it is to hire someone to fill it, and even when you do, communication problems may persist.
See these articles on Forbes.com for definitions of a data scientist from leading experts in the field:
- What is a Data Scientist?: Michael Rappa, NC State University
- IBM’s Anjul Bhambhri on “What Is a Data Scientist?”
- TIBCO Spotfire’s Michael O’Connell on “What is a Data Scientist?”
- Tableau Software’s Pat Hanrahan on “What is a Data Scientist?”
- LinkedIn’s Monica Rogati on “What is a Data Scientist?”
- LinkedIn’s Daniel Tunkelang on “What is a Data Scientist?”
- EMC Greenplum’s Steven Hillion on “What Is a Data Scientist?”
- Amazon’s John Rauser on “What Is a Data Scientist?”
This problem statement addresses the challenge of “growing your own” data scientist. We strongly believe that the person who understands how to make the best use of data is very important. We also believe these people will have to be “created,” rather than hired.
Often, the solution will not be to create a just one person who can be the data scientist, but rather to open up communication so that a team can do the job instead of having to have a virtuoso.
Context and Background
Many sources of data are coming becoming available in almost every dimension of life and business. Vendors are stepping up to the plate by providing tools to understand big data and any other kind of data.
Companies like Splunk and 1010data offer Agile Big Data technology that is simple enough for normal humans to use but powerful enough to handle massive volumes of data. Revolution Analytics aims to make advanced statistics easy to use by enhancing the R suite of statistical software.
Visualization technologies like QlikView, Tableau, and TIBCO Spotfire, are bringing new analytical power to the edges of the organization. These technologies are growing in power and becoming very sophisticated, and are leading us down the path to a world of “user-driven innovation,” where the analysis of complex data is no longer a months-long project for IT, but a quick set of clicks by an inquisitive business user, who can then immediately take action.
In this world of user-driven innovation, how can we bring in the skills to analyze this data into the business world, so people can analyze the data themselves? When the knowledge of the business domain and the knowledge of how to analyze data using advanced techniques are present in one mind, a data scientist is born.
There are three ways to grow a data scientist in most business environments:
- Provide the business staff with tools so they can analyze data and answer questions on their own.
- Communicate the questions that need to be answered by the analytics and IT experts who can then use the advanced technology to answer them.
- Improve communication so that business staff along with the analytics and IT experts can work as a team.
All three approaches are needed. Some technology is empowering and can allow strategy 1 to work. But many valuable ways of analyzing data is too hard for even super users to use, requiring strategy 2.
Ideally, both strategies are in place at the same time, which usually leads to the team mentioned in strategy 3. As the payoff from data becomes more and more clear, the task of growing a data scientist will become urgent. It is unlikely that enough trained data scientists will come out of universities.